Tutorial para gerar o mapa de distribuição de 𝑀𝑎𝑐𝑟𝑜𝑙𝑜𝑏𝑖𝑢𝑚 𝑎𝑟𝑎𝑐𝑎𝑒𝑛𝑠𝑒 Farroñay
By Ricardo de Oliveira Perdiz in mapa R Tutorial
September 1, 2019
Para executar o tutorial abaixo e reproduzir o mapa publicado em Farroñay et al. (2018), é necessário baixar os dados presentes na página
https://github.com/ricoperdiz/Tutorials/tree/master/R_map_2018_Farronayetal. Baixe todos os arquivos, com exceção dos arquivos .r
e .ipynb
(Jupyter Notebook).
Baixe o pdf na seção de
publicações.
01 Carrega os pacotes
Para manipular os dados e gerar o mapa desta postagem, fiz uso dos pacotes abaixo:
- broom (Robinson, Hayes, e Couch 2022);
- cowplot (Wilke 2020);
- dplyr (Wickham et al. 2021);
- ggsn (Santos Baquero 2019);
- GISTools (Brunsdon e Chen 2014);
- magrittr (Bache e Wickham 2020);
- measurements (Birk 2019);
- purrr (Henry e Wickham 2020);
- readr (Wickham, Hester, e Bryan 2021);
- rgdal (Bivand, Keitt, e Rowlingson 2021);
- sf (Pebesma 2021);
- stringr (Wickham 2019).
Tenha certeza de que todos os pacotes listados abaixo estão instalados.
Atenção especial deve ser dada ao pacote ggmap
, que agora requer que seja instalado via GitHub. Veja mais detalhes
aqui. Para isso, instale a versão ggmap
do GitHub rodando o comando abaixo:
# Rode o comando abaixo para instalar a versao do ggmap do GitHub
# Install ggmap package from GitHub version - this is needed in order to be able to download Google Maps nowadays
# if(!requireNamespace("remotes")) {
# install.packages("remotes")
# remotes::install_github("dkahle/ggmap")
# }
# ----------
## Atencao
# ----------
# ---------------------------------------------------------------
## Todos os dados necessarios para plotar o mapa desta publicacao estao disponiveis em:
# <https://github.com/ricoperdiz/Tutorials/tree/master/R_map_2018_Farronayetal>
## Script author: Ricardo Perdiz <ricardoperdiz@yahoo.com>
## Qualquer duvida ou erro, por favor deixe um alerta em <github.com/ricoperdiz/Tutorials/> ou escreva um email.
# ---------------------------------------------------------------
# lista de pacotes necessarios para rodar este tutorial
package_list <- c("dplyr", "stringr", "readr", "purrr", "magrittr", "broom", "rgdal", "measurements", "GISTools", "ggsn", "cowplot", "sf")
# Rode o comando abaixo para instalar todos os pacotes dentro do vetor package_list
# install.packages(package_list, dependencies = TRUE)
# Carregue os pacotes
libs <- c(package_list, "ggmap")
sapply(libs, library, character.only = TRUE, logical.return = TRUE, quietly = TRUE, warn.conflicts = FALSE)
## Warning: package 'broom' was built under R version 4.1.2
## rgdal: version: 1.5-23, (SVN revision 1121)
## Geospatial Data Abstraction Library extensions to R successfully loaded
## Loaded GDAL runtime: GDAL 3.2.1, released 2020/12/29
## Path to GDAL shared files: /Library/Frameworks/R.framework/Versions/4.1/Resources/library/rgdal/gdal
## GDAL binary built with GEOS: TRUE
## Loaded PROJ runtime: Rel. 7.2.1, January 1st, 2021, [PJ_VERSION: 721]
## Path to PROJ shared files: /Library/Frameworks/R.framework/Versions/4.1/Resources/library/rgdal/proj
## PROJ CDN enabled: FALSE
## Linking to sp version:1.4-5
## To mute warnings of possible GDAL/OSR exportToProj4() degradation,
## use options("rgdal_show_exportToProj4_warnings"="none") before loading rgdal.
## Overwritten PROJ_LIB was /Library/Frameworks/R.framework/Versions/4.1/Resources/library/rgdal/proj
## Checking rgeos availability: TRUE
## Please note that 'maptools' will be retired by the end of 2023,
## plan transition at your earliest convenience;
## some functionality will be moved to 'sp'.
## Warning: package 'MASS' was built under R version 4.1.2
##
## Attaching package: 'MASS'
## The following object is masked from 'package:dplyr':
##
## select
## rgeos version: 0.5-9, (SVN revision 684)
## GEOS runtime version: 3.8.1-CAPI-1.13.3
## Please note that rgeos will be retired by the end of 2023,
## plan transition to sf functions using GEOS at your earliest convenience.
## Linking to sp version: 1.4-6
## Polygon checking: TRUE
## Linking to GEOS 3.8.1, GDAL 3.2.1, PROJ 7.2.1
## Google's Terms of Service: https://cloud.google.com/maps-platform/terms/.
## Please cite ggmap if you use it! See citation("ggmap") for details.
## dplyr stringr readr purrr magrittr broom
## TRUE TRUE TRUE TRUE TRUE TRUE
## rgdal measurements GISTools ggsn cowplot sf
## TRUE TRUE TRUE TRUE TRUE TRUE
## ggmap
## TRUE
# If any of the messages below points FALSE, it means that you do not have that package installed. So, please install it before proceeding with the tutorial.
# if you do not remember how to install a package, use the command below
# install.packages("PackageName", dependencies = TRUE)
Os pacotes blogdown (Xie, Dervieux, e Presmanes Hill 2021), rmarkdown (Xie, Allaire, e Grolemund 2018), e knitr (Xie 2021) foram utilizados tanto para gerar a estrutura deste sítio web quanto para gerar os arquivos .html
que vocês estão lendo neste momento.
02 Lê os dados
Dados para este tutorial se dividem entre dados dos espécimes e dados SIG. Dados de espécimes contêm coordenadas, enquanto dados SIG contêm todos os shapefiles necessários para os mapas.
Dados de espécimes
# Macrolobium longipes
nybg <- read_delim("https://raw.githubusercontent.com/ricoperdiz/Tutorials/master/R_map_2018_Farronayetal/nybg.csv", delim = ",")
## Warning: One or more parsing issues, see `problems()` for details
## Rows: 4 Columns: 33
## ── Column specification ────────────────────────────────────────────────────────
## Delimiter: ","
## chr (20): AdmGUIDValue_tab, DarInstitutionCode, DarDatasetName, DarBasisOfRe...
## dbl (6): DarCatalogNumber, DarCollector, DarYearCollected, DarDecimalLatitu...
## lgl (7): DarMinimumElevation, DarMaximumElevation, DarGeorefMethod, DarSubs...
##
## ℹ Use `spec()` to retrieve the full column specification for this data.
## ℹ Specify the column types or set `show_col_types = FALSE` to quiet this message.
splink <- read_delim("https://raw.githubusercontent.com/ricoperdiz/Tutorials/master/R_map_2018_Farronayetal/splink.csv", delim = ";")
## Rows: 4 Columns: 76
## ── Column specification ────────────────────────────────────────────────────────
## Delimiter: ";"
## chr (22): institutioncode, kingdom, descrindividuo, aux_nomecompunidgeo_inv...
## dbl (9): codcolbot, codespecime, numtombo, numcoleta, diacoleta, mescoleta...
## lgl (43): basisofrecord, phylum, class, ordem, fieldnumber, julianday, time...
## date (2): datelastmodified, dataultalter
##
## ℹ Use `spec()` to retrieve the full column specification for this data.
## ℹ Specify the column types or set `show_col_types = FALSE` to quiet this message.
inpa <- read_delim("https://raw.githubusercontent.com/ricoperdiz/Tutorials/master/R_map_2018_Farronayetal/inpa.csv", delim = ",")
## Rows: 2 Columns: 5
## ── Column specification ────────────────────────────────────────────────────────
## Delimiter: ","
## chr (4): COORD_, HERBARIA, COLETOR, ESPECIE
## dbl (1): NUMERO_COL
##
## ℹ Use `spec()` to retrieve the full column specification for this data.
## ℹ Specify the column types or set `show_col_types = FALSE` to quiet this message.
# Macrolobium aracaense
araca <- read_delim("https://raw.githubusercontent.com/ricoperdiz/Tutorials/master/R_map_2018_Farronayetal/Maracaense.csv", delim = "\t")
## Rows: 2 Columns: 17
## ── Column specification ────────────────────────────────────────────────────────
## Delimiter: "\t"
## chr (10): COLETOR, ADDCOLL, COUNTRY, MINORAREA, MAJORAREA, GAZETTER, COORDEN...
## dbl (6): NUMERO, COLDD, COLM, COLY, LATITUDE, LONGITUDE
## lgl (1): SP1
##
## ℹ Use `spec()` to retrieve the full column specification for this data.
## ℹ Specify the column types or set `show_col_types = FALSE` to quiet this message.
Dados SIG
# South and Central America shape
area_mapa <- rgdal::readOGR(dsn = "SAm_CAm_shape.shp")
## OGR data source with driver: ESRI Shapefile
## Source: "/Users/ricoperdiz/Documents/PROFISSIONAL/Sites/ricardoperdiz/content/blog/2020_03_29_R-map-Farronayetal-2018/SAm_CAm_shape.shp", layer: "SAm_CAm_shape"
## with 37 features
## It has 65 fields
# Brazilian states shapefile
estados <- st_read("BRASIL.shp")
## Reading layer `BRASIL' from data source
## `/Users/ricoperdiz/Documents/PROFISSIONAL/Sites/ricardoperdiz/content/blog/2020_03_29_R-map-Farronayetal-2018/BRASIL.shp'
## using driver `ESRI Shapefile'
## Simple feature collection with 27 features and 3 fields
## Geometry type: MULTIPOLYGON
## Dimension: XY
## Bounding box: xmin: -73.83943 ymin: -33.77086 xmax: -34.8581 ymax: 5.38289
## CRS: NA
# Filtra apenas o estado do Amazonas dentro do objeto `estados`
br_amazonas <- estados %>% filter(ESTADO == "Amazonas")
br_amazonas
## Simple feature collection with 1 feature and 3 fields
## Geometry type: MULTIPOLYGON
## Dimension: XY
## Bounding box: xmin: -73.68224 ymin: -9.847688 xmax: -55.99345 ymax: 2.198446
## CRS: NA
## UF ESTADO REGIAO geometry
## 1 AM Amazonas NO MULTIPOLYGON (((-63.47887 2...
br_amazonas_tidy <- st_geometry(br_amazonas)
br_amazonas_tidy
## Geometry set for 1 feature
## Geometry type: MULTIPOLYGON
## Dimension: XY
## Bounding box: xmin: -73.68224 ymin: -9.847688 xmax: -55.99345 ymax: 2.198446
## CRS: NA
## MULTIPOLYGON (((-63.47887 2.198446, -63.43955 2...
area_mapa_tidy <- tidy(area_mapa)
## Regions defined for each Polygons
area_mapa_tidy
## # A tibble: 61,879 × 7
## long lat order hole piece group id
## <dbl> <dbl> <int> <lgl> <fct> <fct> <chr>
## 1 -70.0 12.6 1 FALSE 1 0.1 0
## 2 -69.9 12.5 2 FALSE 1 0.1 0
## 3 -69.9 12.5 3 FALSE 1 0.1 0
## 4 -69.9 12.5 4 FALSE 1 0.1 0
## 5 -69.9 12.5 5 FALSE 1 0.1 0
## 6 -69.9 12.4 6 FALSE 1 0.1 0
## 7 -69.9 12.4 7 FALSE 1 0.1 0
## 8 -69.9 12.4 8 FALSE 1 0.1 0
## 9 -69.9 12.4 9 FALSE 1 0.1 0
## 10 -69.9 12.4 10 FALSE 1 0.1 0
## # … with 61,869 more rows
03 Limpa os dados
Primeiro, limparemos os dados da espécie Macrolobium longipes R.S. Cowan.
# Macrolobium longipes
# first data from inpa
inpa_modif <- inpa %>%
mutate(
lat = str_split(COORD_, "N") %>%
sapply("[[", 1) %>%
gsub("°", " ", .) %>%
gsub("’", " ", .) %>%
paste0(., " 0") %>%
gsub(" | ", " ", .) %>%
str_trim("both") %>%
measurements::conv_unit(., from = "deg_min_sec", to = "dec_deg"),
long = str_split(COORD_, "N") %>%
sapply("[[", 2) %>%
gsub("°", " ", .) %>%
gsub("’", " ", .) %>%
gsub("W", "0", .) %>%
str_trim("both") %>%
gsub(" ", " ", .) %>%
measurements::conv_unit(., from = "deg_min_sec", to = "dec_deg")
) %>%
dplyr::select(-COORD_)
inpa_modif
## # A tibble: 2 × 6
## HERBARIA COLETOR NUMERO_COL ESPECIE lat long
## <chr> <chr> <dbl> <chr> <chr> <chr>
## 1 INPA Huber 5849 Macrolobium longipes 1.58333333333333 66.55
## 2 INPA Rodrigues 10875 Macrolobium longipes 0.333333333333333 67.31666…
inpa_modif %>% glimpse()
## Rows: 2
## Columns: 6
## $ HERBARIA <chr> "INPA", "INPA"
## $ COLETOR <chr> "Huber", "Rodrigues"
## $ NUMERO_COL <dbl> 5849, 10875
## $ ESPECIE <chr> "Macrolobium longipes", "Macrolobium longipes"
## $ lat <chr> "1.58333333333333", "0.333333333333333"
## $ long <chr> "66.55", "67.3166666666667"
names(inpa_modif) <- tolower(names(inpa_modif)) # turn all column names as lower case
names(inpa_modif)
## [1] "herbaria" "coletor" "numero_col" "especie" "lat"
## [6] "long"
# then we get speciesLink data and select only columns we want to keep
longipes <- splink %>%
dplyr::select(
herbaria = institutioncode,
coletor, numero_col = numcoleta,
especie = taxoncompleto,
pais, estado = estado_prov,
local = descrlocal
) %>%
# then join them with data from INPA
full_join(inpa_modif, .) %>%
dplyr::select(-estado) %>%
# turn lat and long columns as numeric
mutate_at(
.vars = c("lat", "long"),
.funs = as.numeric
)
## Joining, by = c("herbaria", "coletor", "numero_col", "especie")
longipes
## # A tibble: 6 × 8
## herbaria coletor numero_col especie lat long pais local
## <chr> <chr> <dbl> <chr> <dbl> <dbl> <chr> <chr>
## 1 INPA Huber 5849 Macrolobium… 1.58 66.6 <NA> <NA>
## 2 INPA Rodrigues 10875 Macrolobium… 0.333 67.3 <NA> <NA>
## 3 JBRJ Maguire, B. 37590 Macrolobium… NA NA Venez… "Rio Siapa, …
## 4 JBRJ Maguire, B. 37572 Macrolobium… NA NA Venez… "Rio Siapa, …
## 5 JBRJ Maguire, B. 37643 Macrolobium… NA NA Venez… "Rio Siapa, …
## 6 JBRJ Maguire, B. 37590 Macrolobium… NA NA Venez… "Rio Siapa, …
longipes %>% glimpse()
## Rows: 6
## Columns: 8
## $ herbaria <chr> "INPA", "INPA", "JBRJ", "JBRJ", "JBRJ", "JBRJ"
## $ coletor <chr> "Huber", "Rodrigues", "Maguire, B.", "Maguire, B.", "Maguir…
## $ numero_col <dbl> 5849, 10875, 37590, 37572, 37643, 37590
## $ especie <chr> "Macrolobium longipes", "Macrolobium longipes", "Macrolobiu…
## $ lat <dbl> 1.5833333, 0.3333333, NA, NA, NA, NA
## $ long <dbl> 66.55000, 67.31667, NA, NA, NA, NA
## $ pais <chr> NA, NA, "Venezuela", "Venezuela", "Venezuela", "Venezuela"
## $ local <chr> NA, NA, "Rio Siapa, Casiquiare, Territorio Amazonas. ", "Ri…
# at last, we get nybg data and select and rename columns that we want to keep
nybg_modif <- nybg %>%
dplyr::select(
herbaria = DarInstitutionCode,
coletor = DarCollectorNumber,
numero_col = DarCollector,
local = DarLocality,
lat = DarDecimalLatitude,
long = DarDecimalLongitude,
pais = DarCountry
)
nybg_modif
## # A tibble: 4 × 7
## herbaria coletor numero_col local lat long pais
## <chr> <chr> <dbl> <chr> <dbl> <dbl> <chr>
## 1 NY H. C. de Lima 3279 Alto Rio Negro, Rio U… -0.130 -67.1 Brazil
## 2 NY B. Maguire 37590 On right bank of Río … NA NA Venez…
## 3 NY B. Maguire 37590 On right bank of Río … NA NA Venez…
## 4 NY W. A. Rodrigues 10875 Alto Rio Negro. Igara… 0.333 -67.3 Brazil
Sempre é bom olhar nossos dados, checar o tipo de variável para cada coluna, quantas observações e quantas variáveis temos em nossos dados.
Podemos fazer isso utilizando a função str()
do pacote base do R, ou a função glimpse
do pacote dplyr.
Aqui usarei a função glimpse
no conjunto de dados longipes
.
glimpse(longipes)
## Rows: 6
## Columns: 8
## $ herbaria <chr> "INPA", "INPA", "JBRJ", "JBRJ", "JBRJ", "JBRJ"
## $ coletor <chr> "Huber", "Rodrigues", "Maguire, B.", "Maguire, B.", "Maguir…
## $ numero_col <dbl> 5849, 10875, 37590, 37572, 37643, 37590
## $ especie <chr> "Macrolobium longipes", "Macrolobium longipes", "Macrolobiu…
## $ lat <dbl> 1.5833333, 0.3333333, NA, NA, NA, NA
## $ long <dbl> 66.55000, 67.31667, NA, NA, NA, NA
## $ pais <chr> NA, NA, "Venezuela", "Venezuela", "Venezuela", "Venezuela"
## $ local <chr> NA, NA, "Rio Siapa, Casiquiare, Territorio Amazonas. ", "Ri…
glimpse(nybg_modif)
## Rows: 4
## Columns: 7
## $ herbaria <chr> "NY", "NY", "NY", "NY"
## $ coletor <chr> "H. C. de Lima", "B. Maguire", "B. Maguire", "W. A. Rodrigu…
## $ numero_col <dbl> 3279, 37590, 37590, 10875
## $ local <chr> "Alto Rio Negro, Rio Uaupés, Igarapé Tibuarí, acima do Seri…
## $ lat <dbl> -0.130278, NA, NA, 0.333333
## $ long <dbl> -67.0892, NA, NA, -67.3167
## $ pais <chr> "Brazil", "Venezuela", "Venezuela", "Brazil"
longipes <-
longipes %>%
full_join(., nybg_modif)
## Joining, by = c("herbaria", "coletor", "numero_col", "lat", "long", "pais", "local")
longipes # now with data from INPA and NY herbaria
## # A tibble: 10 × 8
## herbaria coletor numero_col especie lat long pais local
## <chr> <chr> <dbl> <chr> <dbl> <dbl> <chr> <chr>
## 1 INPA Huber 5849 Macrolob… 1.58 66.6 <NA> <NA>
## 2 INPA Rodrigues 10875 Macrolob… 0.333 67.3 <NA> <NA>
## 3 JBRJ Maguire, B. 37590 Macrolob… NA NA Venez… "Rio Siapa…
## 4 JBRJ Maguire, B. 37572 Macrolob… NA NA Venez… "Rio Siapa…
## 5 JBRJ Maguire, B. 37643 Macrolob… NA NA Venez… "Rio Siapa…
## 6 JBRJ Maguire, B. 37590 Macrolob… NA NA Venez… "Rio Siapa…
## 7 NY H. C. de Lima 3279 <NA> -0.130 -67.1 Brazil "Alto Rio …
## 8 NY B. Maguire 37590 <NA> NA NA Venez… "On right …
## 9 NY B. Maguire 37590 <NA> NA NA Venez… "On right …
## 10 NY W. A. Rodrigues 10875 <NA> 0.333 -67.3 Brazil "Alto Rio …
longipes <-
longipes %>%
filter(!is.na(lat), coletor != "Rodrigues") %>%
# add species name in a separate column
mutate(
especie = "M. longipes"
)
glimpse(longipes)
## Rows: 3
## Columns: 8
## $ herbaria <chr> "INPA", "NY", "NY"
## $ coletor <chr> "Huber", "H. C. de Lima", "W. A. Rodrigues"
## $ numero_col <dbl> 5849, 3279, 10875
## $ especie <chr> "M. longipes", "M. longipes", "M. longipes"
## $ lat <dbl> 1.583333, -0.130278, 0.333333
## $ long <dbl> 66.5500, -67.0892, -67.3167
## $ pais <chr> NA, "Brazil", "Brazil"
## $ local <chr> NA, "Alto Rio Negro, Rio Uaupés, Igarapé Tibuarí, acima do …
Agora limparemos os dados referentes à espécie nova descrita em Farroñay et al. (2018), chamada Macrolobium aracaense Farroñay.
# Macrolobium aracaense
## Clean data
# turn column names to lower case
names(araca) <- tolower(names(araca))
araca_md <- araca %>%
mutate(
# clean coordinate columns to extract and convert degrees min sec to decimal degrees
lat = str_split(coordenadas, "N") %>%
sapply("[[", 1) %>%
gsub("°", " ", .) %>%
gsub("\'|\'\'|\"", " ", .) %>%
gsub(" | ", " ", .) %>%
str_trim("both") %>%
measurements::conv_unit(., from = "deg_min_sec", to = "dec_deg"),
# do the same done for long data
long = str_split(coordenadas, "N") %>%
sapply("[[", 2) %>%
gsub("W", "", .) %>%
gsub("°", " ", .) %>%
gsub("\'|\'\'|\"", " ", .) %>%
gsub(" | ", " ", .) %>%
str_trim("both") %>%
measurements::conv_unit(., from = "deg_min_sec", to = "dec_deg"),
especie = "M. aracaense"
) %>%
dplyr::select(coletor,
numero_col = numero,
pais = country,
lat, long, estado = majorarea, herbaria, especie
) %>%
# turn lat and long columns as numeric
mutate_at(
.vars = c("lat", "long"),
.funs = as.numeric
)
glimpse(araca_md)
## Rows: 2
## Columns: 8
## $ coletor <chr> "Vicentini, A.", "Farroñay, F."
## $ numero_col <dbl> 1886, 178
## $ pais <chr> "Brasil", "Brasil"
## $ lat <dbl> 0.9483333, 0.8633333
## $ long <dbl> 63.39167, 63.33167
## $ estado <chr> "Amazonas", "Amazonas"
## $ herbaria <chr> "INPA", "INPA"
## $ especie <chr> "M. aracaense", "M. aracaense"
# join data for Macrolobium longipes and M. aracaense
dados <-
full_join(longipes, araca_md) %>%
dplyr::select(herbaria, coletor, numero_col, especie, lat, long)
## Joining, by = c("herbaria", "coletor", "numero_col", "especie", "lat", "long", "pais")
glimpse(dados)
## Rows: 5
## Columns: 6
## $ herbaria <chr> "INPA", "NY", "NY", "INPA", "INPA"
## $ coletor <chr> "Huber", "H. C. de Lima", "W. A. Rodrigues", "Vicentini, A.…
## $ numero_col <dbl> 5849, 3279, 10875, 1886, 178
## $ especie <chr> "M. longipes", "M. longipes", "M. longipes", "M. aracaense"…
## $ lat <dbl> 1.5833333, -0.1302780, 0.3333330, 0.9483333, 0.8633333
## $ long <dbl> 66.55000, -67.08920, -67.31670, 63.39167, 63.33167
dados$long <- ifelse(dados$long > 0, dados$long * -1, dados$long)
longipes <- dados %>%
filter(especie == "M. longipes")
aracaense <- dados %>%
filter(especie == "M. aracaense")
Agora temos dois objetos contendo os dados necessários para cada espécie, Macrolobium longipes e M. aracaense.
longipes
## # A tibble: 3 × 6
## herbaria coletor numero_col especie lat long
## <chr> <chr> <dbl> <chr> <dbl> <dbl>
## 1 INPA Huber 5849 M. longipes 1.58 -66.6
## 2 NY H. C. de Lima 3279 M. longipes -0.130 -67.1
## 3 NY W. A. Rodrigues 10875 M. longipes 0.333 -67.3
aracaense
## # A tibble: 2 × 6
## herbaria coletor numero_col especie lat long
## <chr> <chr> <dbl> <chr> <dbl> <dbl>
## 1 INPA Vicentini, A. 1886 M. aracaense 0.948 -63.4
## 2 INPA Farroñay, F. 178 M. aracaense 0.863 -63.3
04 Cria variáveis para o mapa
# longitude range - main map
x1 <- st_bbox(br_amazonas)[c(1, 3)]
x1
## xmin xmax
## -73.68224 -55.99345
y1 <- st_bbox(br_amazonas)[c(2, 4)]
y1
## ymin ymax
## -9.847688 2.198446
# longitude and latitude range - for ggmap
lat_long <- dados
y_geral <- range(lat_long$lat) + c(-0.01, 0.01)
y_geral
## [1] -0.140278 1.593333
x_geral <- range(lat_long$long) + c(-0.01, 0.01)
x_geral
## [1] -67.32670 -63.32167
05 Baixa o mapa via pacote R ggmap
Recentemente, houve uma mudança na maneira de baixar mapas Google através do pacote ggmap
. Agora é necessária a obtenção de uma chave Google e utiizá-la ao rodar os comandos abaixo com a função mostrada abaixo. Após ese passo, o usuário terá a permissão de baixar os mapas Google usando a função ggmap::get_map
.
Para mais informações, visite este sítio web.
# Place your google KEY here - without it, you WILL NOT be able to download a google map
# register_google(key = 'PlaceYourKeyRightHERE!')
# local_mp <- c(lon = sum(x_geral)/2, lat = sum(y_geral)/2)
# local_mp
# mapa <- get_map(location = local_mp,
# source = "google",
# maptype = "terrain", crop = FALSE,
# zoom = 7,
# color = 'bw',
# language = 'en-EN')
Para checar se funcionou, tente plotar o objeto mapa
:
mapa
Se você não obteve sucesso em baixar o mapa, utilize o arquivo .RDS abaixo para poder prosseguir com o tutorial. Ele contem exatamente o mesmo mapa baixado do Google utilizado na publicação, e se encontra disponível na pasta https://github.com/ricoperdiz/Tutorials/tree/master/R_map_2018_Farronayetal.
mapa <- readRDS("Farronayetal2017_ggmap.RDS")
ggmap(mapa, extent = "panel")
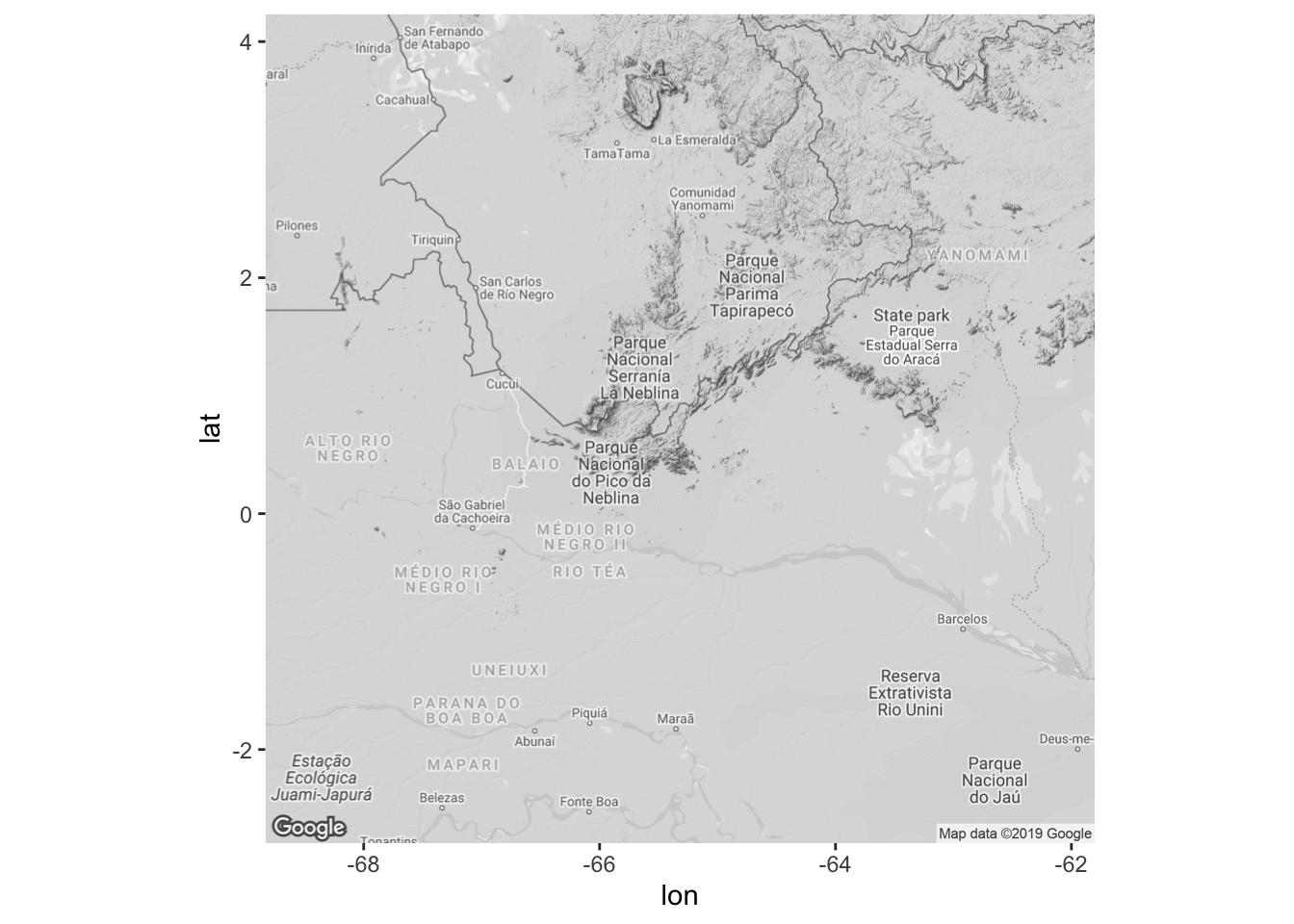
06 Plotam-se os mapas
# Main map - species distribution
x_escala <- -65.1
araca_map1 <-
ggmap(mapa, extent = "panel") +
xlab("Longitude (WGS84)") +
ylab("Latitude")
# plot the map with X and Y Label on it
# take a first look to see if everything is all right
araca_map1
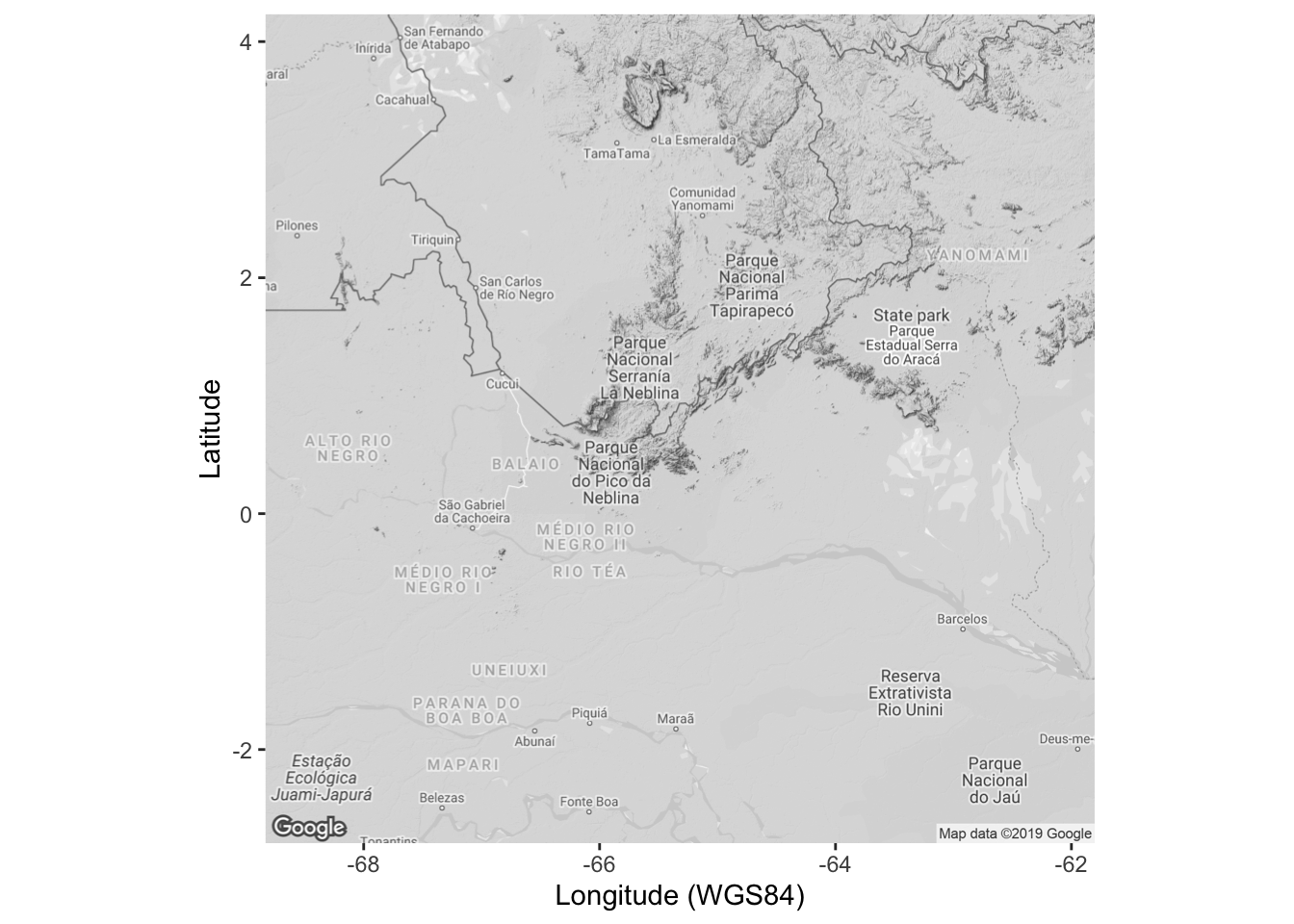
# Main map
araca_map <-
araca_map1 +
geom_point(aes(x = long, y = lat, shape = especie), data = dados, alpha = .8, color = "black", size = 4) +
# add scale bar on topleft
scalebar(
x.min = attr(mapa, "bb")[[2]],
y.min = attr(mapa, "bb")[[1]],
x.max = attr(mapa, "bb")[[4]],
y.max = attr(mapa, "bb")[[3]],
dist = 100, anchor = c(x = -66, y = -2.2),
model = "WGS84",
location = "topleft",
st.size = 4,
st.dist = 0.02,
transform = TRUE,
dist_unit = "km"
) +
# add North arrow
geom_segment(
arrow = arrow(length = unit(4, "mm"), type = "closed", angle = 40),
aes(x = x_escala, xend = x_escala, y = -1.7, yend = -1.3), colour = "black", size = 2
) +
annotate(
x = x_escala, y = -1.85, label = "N", color = "black", geom = "text", size = 6,
fontface = "bold"
) +
# change ggplot2 theme to Black and White
theme_bw() +
# change few details:
# color contour of panel border, legend position, plot margin etc
theme(
panel.border = element_rect(colour = "black", fill = NA, size = 1),
legend.title = element_blank(), legend.text = element_text(size = 12, face = "bold.italic"),
legend.position = "bottom",
plot.margin = unit(x = c(0.1, 0.1, 0, 0.1), units = "in")
)
araca_map
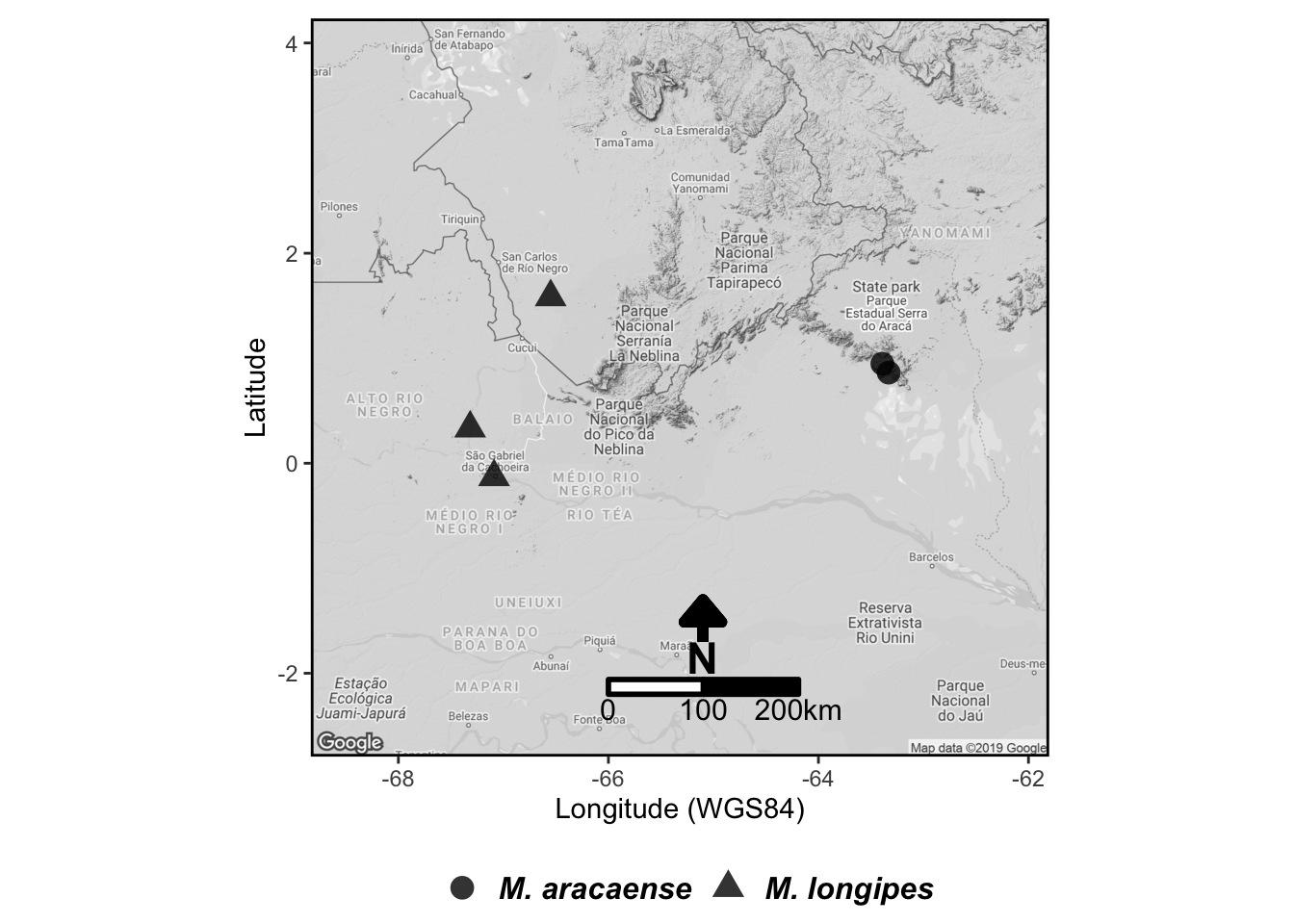
# plot the overview map
overviewmap1 <-
ggplot() +
geom_polygon(
data = as_tibble(st_coordinates(br_amazonas_tidy)),
aes(x = X, y = Y, group = L1),
color = "gray70", fill = "gray70", linetype = 3
)
overviewmap1
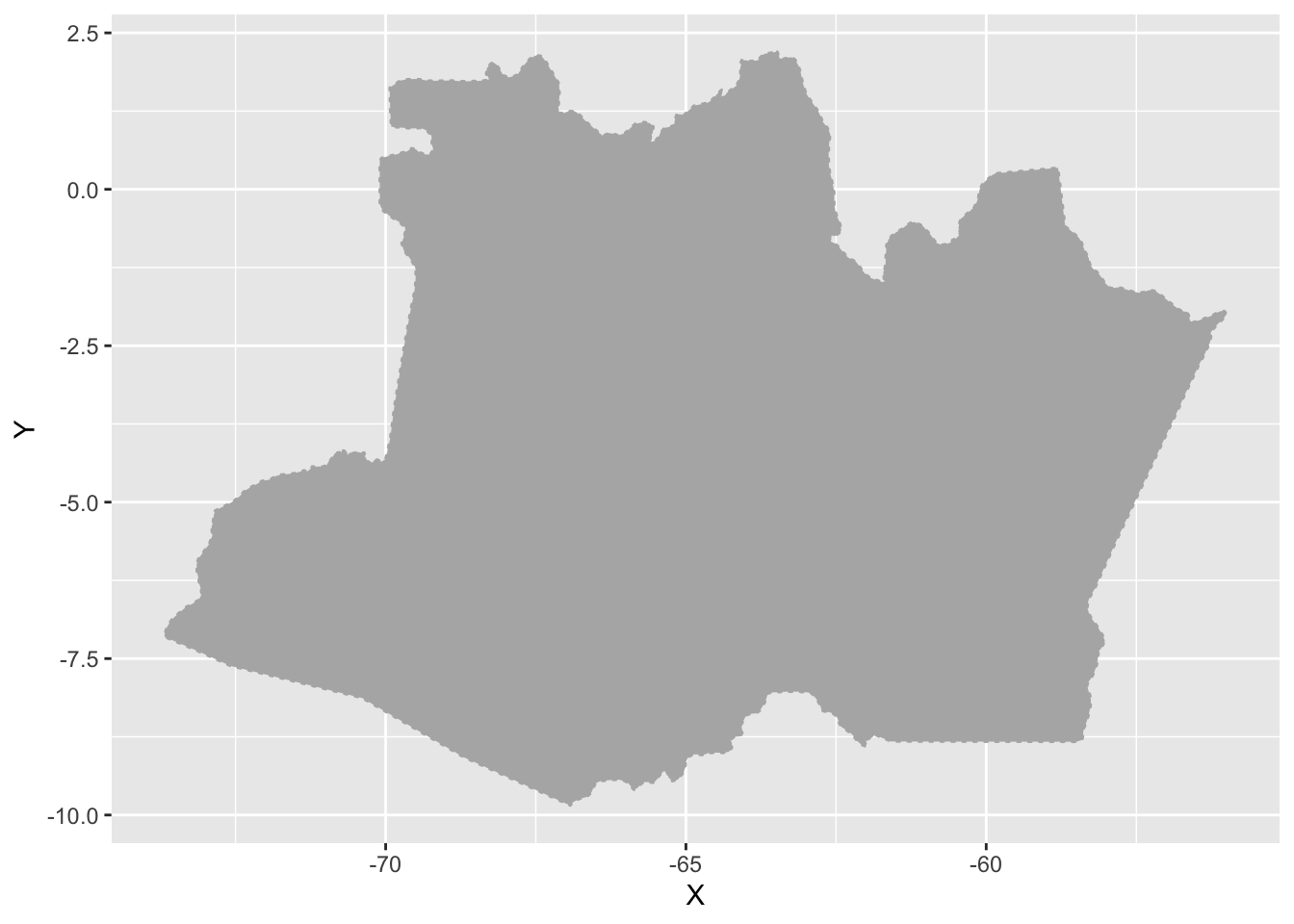
overviewmap2 <- overviewmap1 +
geom_polygon(
data = area_mapa_tidy,
aes(x = long, y = lat, group = group),
color = "black", fill = NA
) +
geom_point(
data = subset(dados, especie %in% "M. aracaense"),
aes(x = long, y = lat), size = 2,
pch = 19, col = "black"
)
overviewmap2
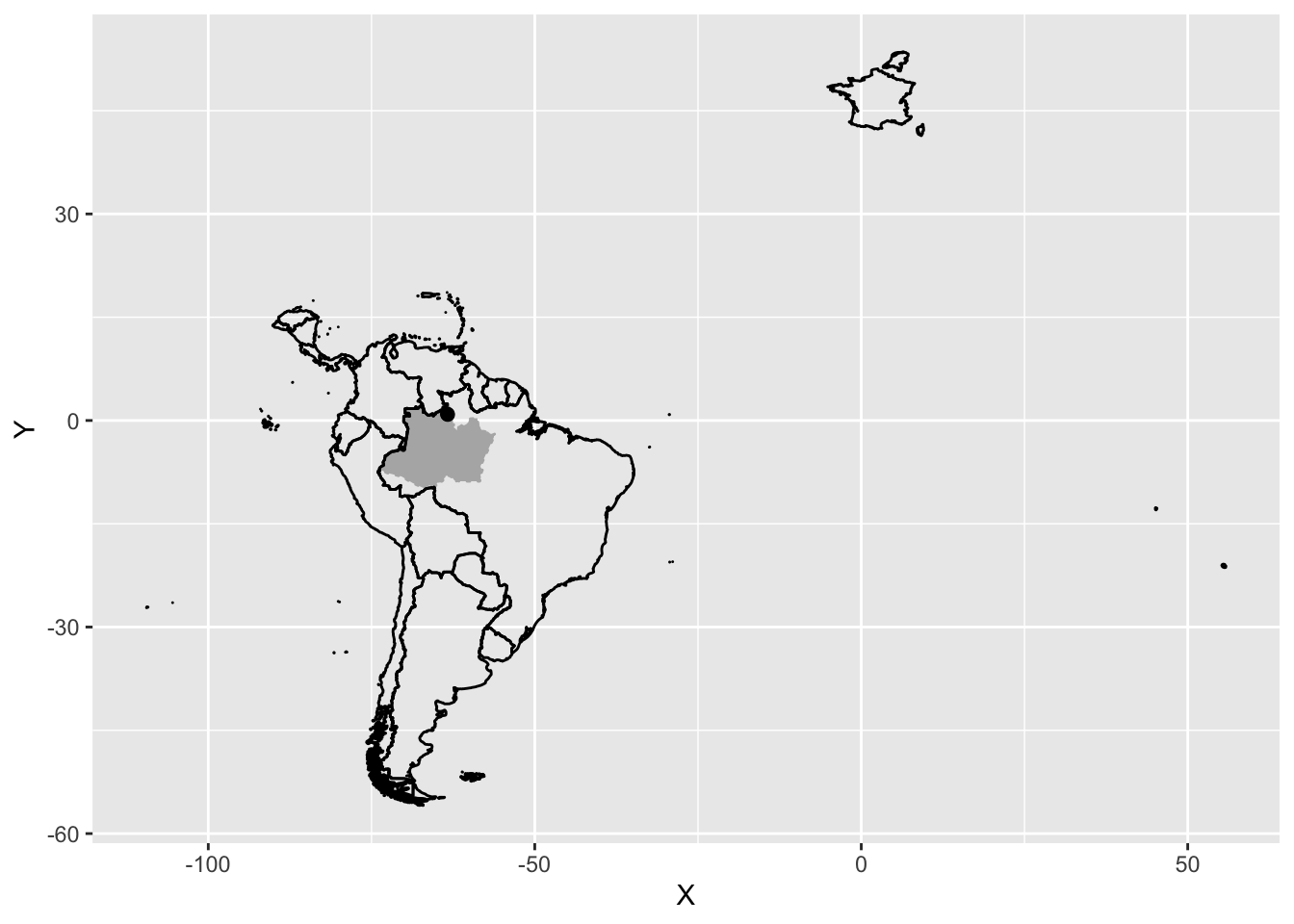
overviewmap3 <- overviewmap2 +
coord_equal() +
coord_cartesian(
xlim = x1 - c(2, -2), ylim = y1 - c(2, -2)
)
## Coordinate system already present. Adding new coordinate system, which will replace the existing one.
overviewmap3
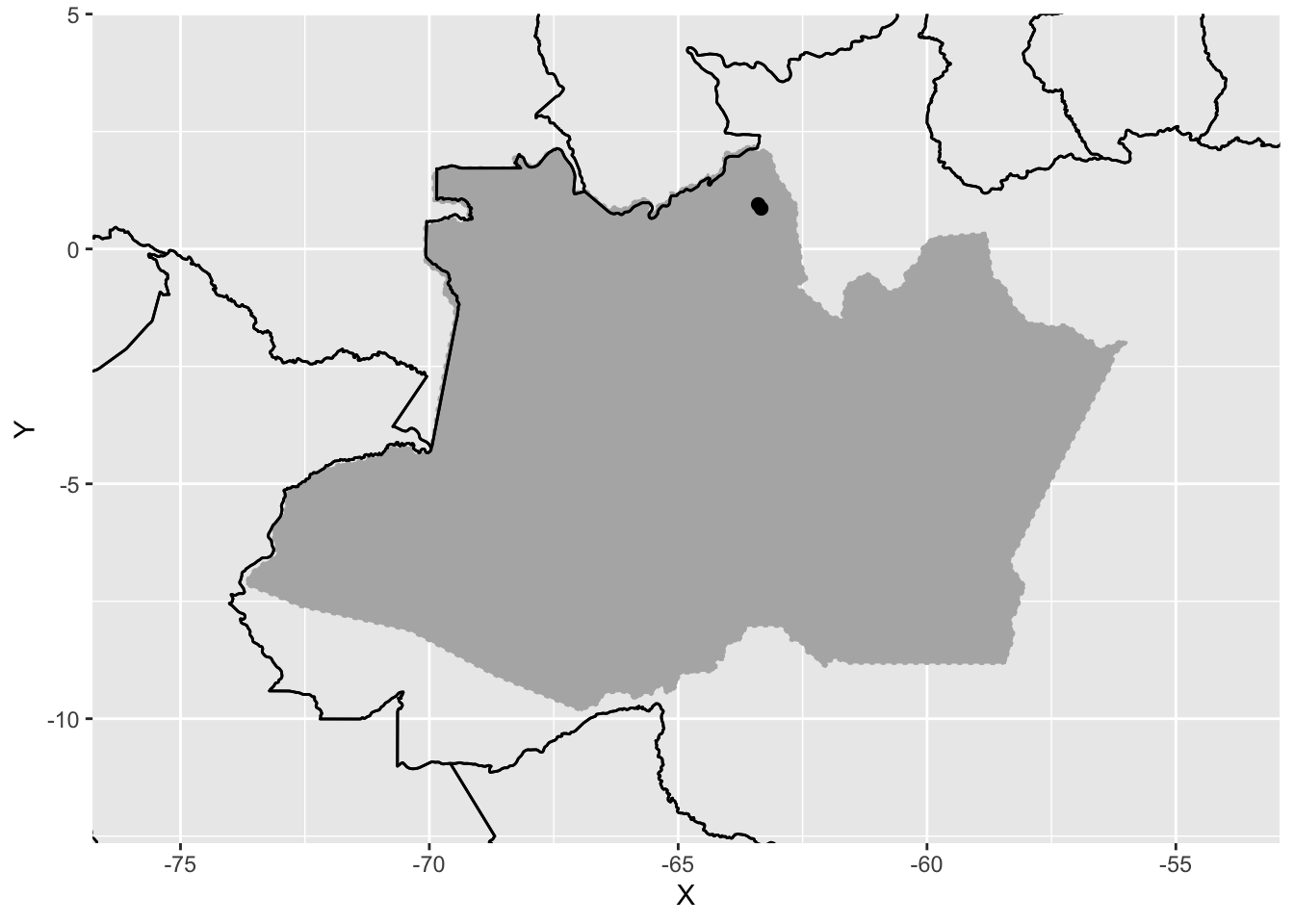
overviewmap <- overviewmap3 +
theme(
plot.background =
element_rect(
fill = "white", linetype = 1,
size = 0.3, colour = "black"
),
axis.line = element_blank(),
axis.text.x = element_blank(),
axis.text.y = element_blank(),
axis.ticks = element_blank(),
axis.title.x = element_blank(),
axis.title.y = element_blank(),
legend.position = "none"
) +
annotate(
x = -63, y = -5, label = "BRAZIL\nAmazonas",
color = "black", geom = "text",
size = 3, fontface = "bold"
)
overviewmap
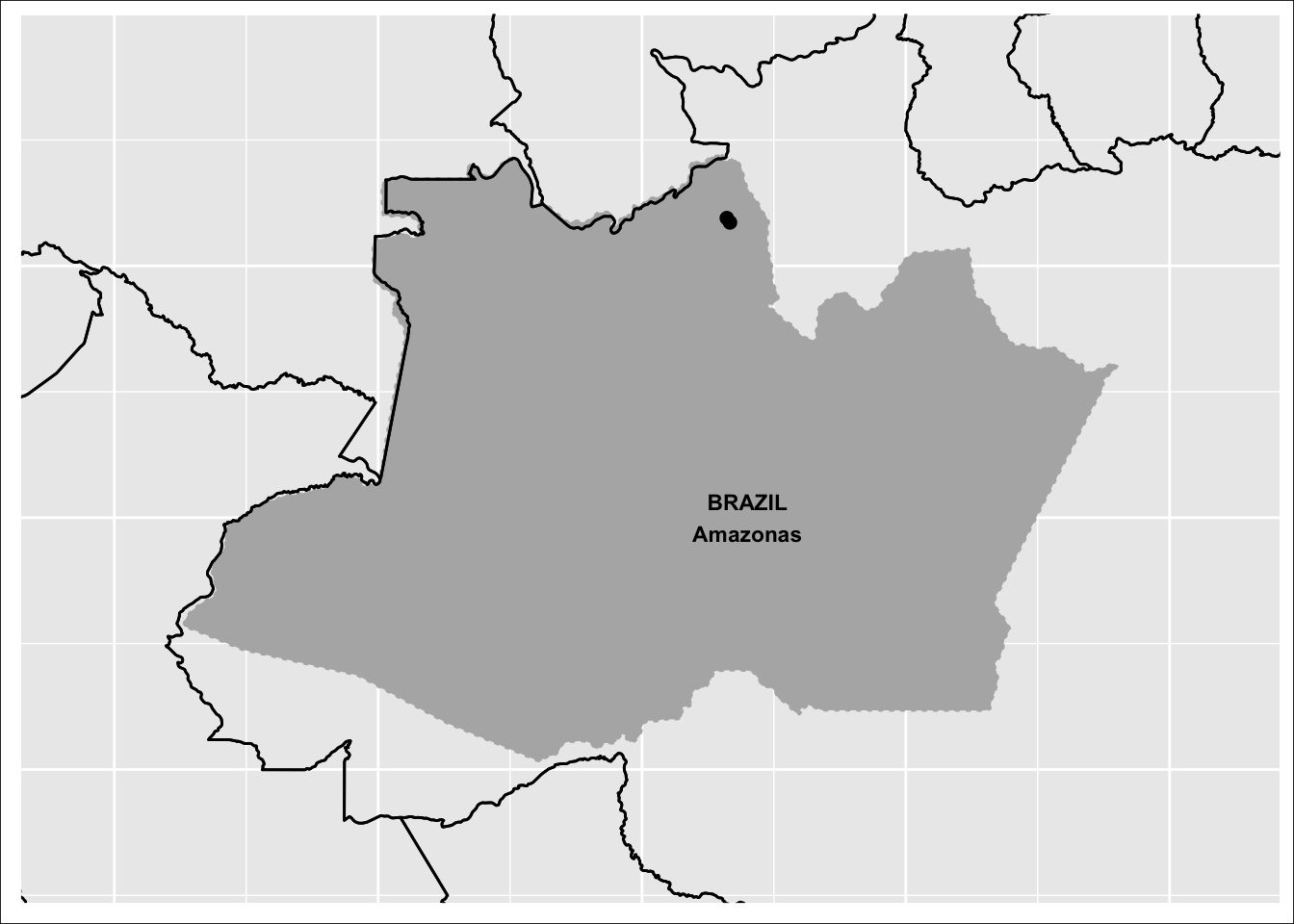
07 Combinam-se os mapas com o pacote R cowplot
final_map <- ggdraw() +
draw_plot(araca_map, 0, 0, 1, 1) +
draw_plot(overviewmap, 0.15, 0.72, 0.225, 0.225)
final_map
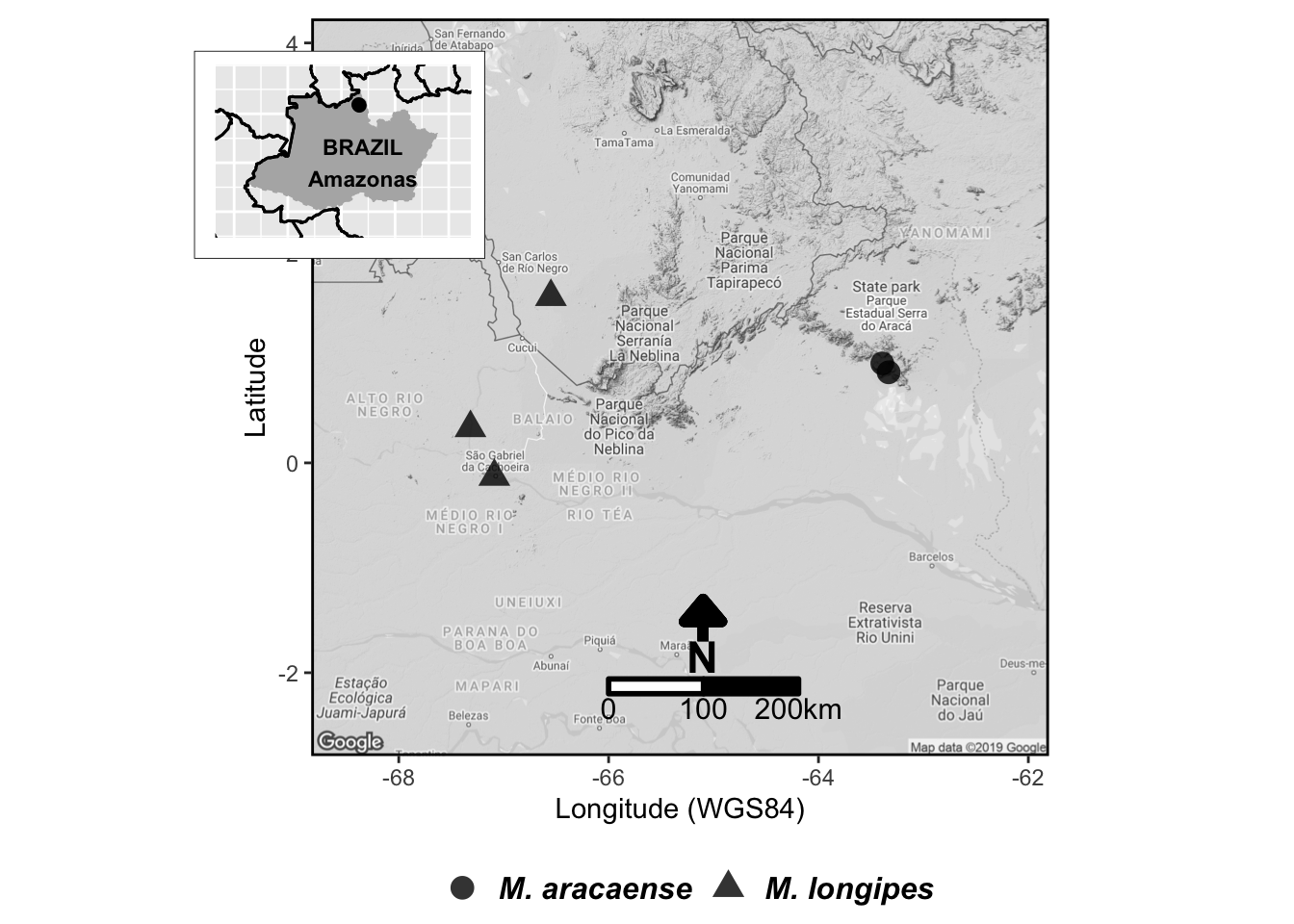
08 Salva o mapa final localmente
cowplot::ggsave2(
plot = final_map,
filename = "final_map_Macrolobium_aracaense_ggmap.pdf", dpi = 600, width = 6, height = 6, units = "in"
)
Compare agora a imagem produzida aqui (veja abaixo!) com a publicada em Farroñay et al. (2018).
Referências
Bache, Stefan Milton, e Hadley Wickham. 2020. magrittr: A Forward-Pipe Operator for R. https://CRAN.R-project.org/package=magrittr.
Birk, Matthew A. 2019. measurements: Tools for Units of Measurement. https://CRAN.R-project.org/package=measurements.
Bivand, Roger, Tim Keitt, e Barry Rowlingson. 2021. rgdal: Bindings for the Geospatial Data Abstraction Library. https://CRAN.R-project.org/package=rgdal.
Brunsdon, Chris, e Hongyan Chen. 2014. GISTools: Some further GIS capabilities for R. https://CRAN.R-project.org/package=GISTools.
Farroñay, Francisco, Marisabel U. Adrianzén, Ricardo Oliveira Perdiz, e Alberto Vicentini. 2018. “A new species of Macrolobium (Fabaceae, Detarioideae) endemic on a Tepui of the Guyana shield in Brazil”. Phytotaxa 361 (1): 97–105. https://doi.org/10.11646/phytotaxa.361.1.8.
Henry, Lionel, e Hadley Wickham. 2020. purrr: Functional Programming Tools. https://CRAN.R-project.org/package=purrr.
Pebesma, Edzer. 2021. sf: Simple Features for R. https://CRAN.R-project.org/package=sf.
Robinson, David, Alex Hayes, e Simon Couch. 2022. broom: Convert Statistical Objects into Tidy Tibbles. https://CRAN.R-project.org/package=broom.
Santos Baquero, Oswaldo. 2019. ggsn: North Symbols and Scale Bars for Maps Created with ggplot2 or ggmap. https://github.com/oswaldosantos/ggsn.
Wickham, Hadley. 2019. stringr: Simple, Consistent Wrappers for Common String Operations. https://CRAN.R-project.org/package=stringr.
Wickham, Hadley, Romain François, Lionel Henry, e Kirill Müller. 2021. dplyr: A Grammar of Data Manipulation. https://CRAN.R-project.org/package=dplyr.
Wickham, Hadley, Jim Hester, e Jennifer Bryan. 2021. readr: Read Rectangular Text Data. https://CRAN.R-project.org/package=readr.
Wilke, Claus O. 2020. cowplot: Streamlined Plot Theme and Plot Annotations for ggplot2. https://wilkelab.org/cowplot/.
Xie, Yihui. 2021. knitr: A General-Purpose Package for Dynamic Report Generation in R. https://yihui.org/knitr/.
Xie, Yihui, J. J. Allaire, e Garrett Grolemund. 2018. R Markdown: The Definitive Guide. Boca Raton, Florida: Chapman; Hall/CRC. https://bookdown.org/yihui/rmarkdown.
Xie, Yihui, Christophe Dervieux, e Alison Presmanes Hill. 2021. blogdown: Create Blogs and Websites with R Markdown. https://CRAN.R-project.org/package=blogdown.
- Posted on:
- September 1, 2019
- Length:
- 19 minute read, 3902 words